Part 2: Binocular Stereo
The goal of this part is to implement a simple window-based stereo matching algorithm
for rectified stereo pairs. You will be using the following stereo pairs:
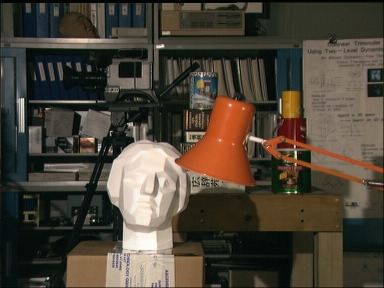
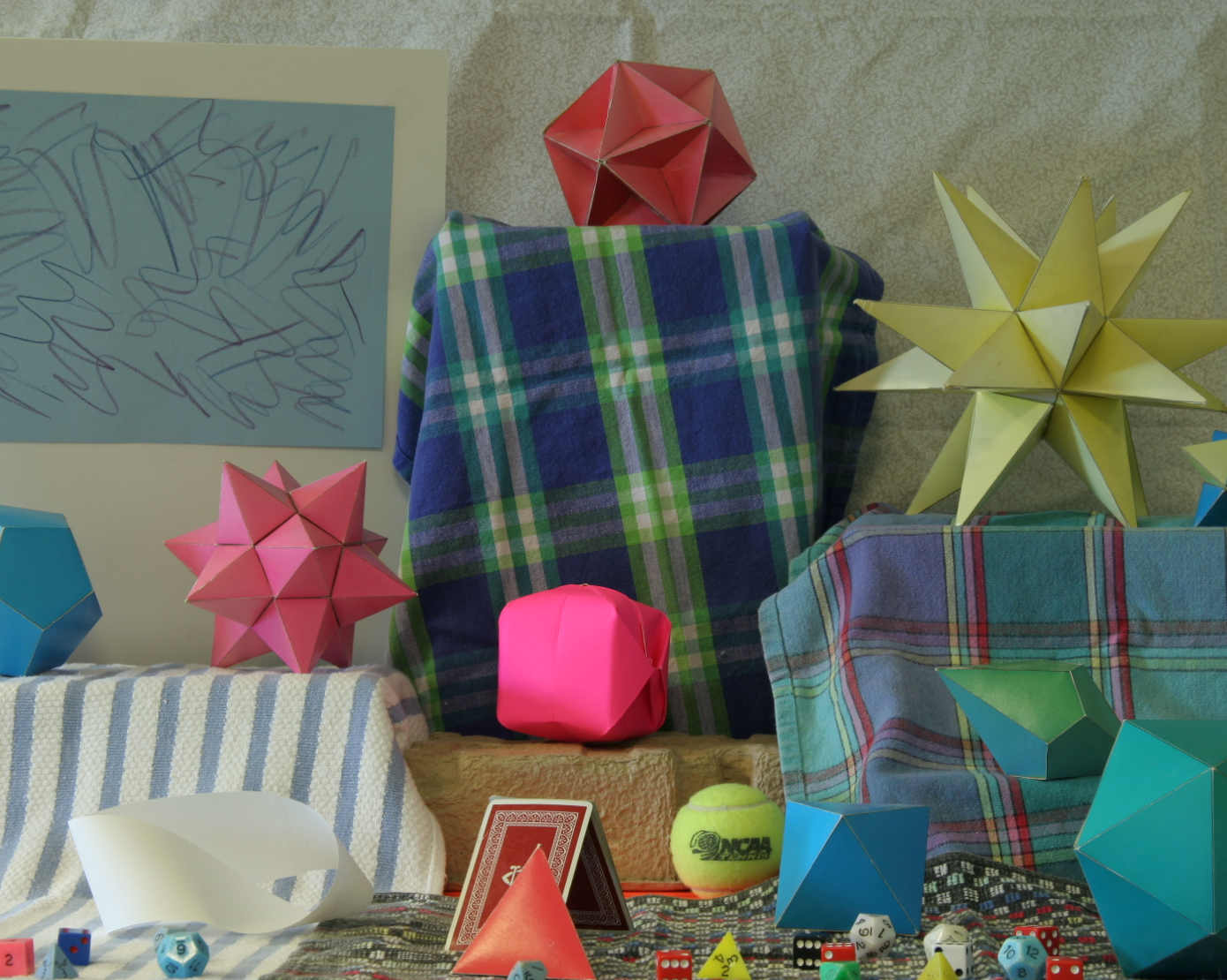
Follow the basic outline given in lecture: pick a window around
each pixel in the first (reference) image, and then search the corresponding scanline in the second
image for a matching window. The output should be a disparity map with respect to the first view
(use these ground truth maps for qualitative reference for first pair and second pair).
You should experiment with the following settings and parameters:
- Search window size: show disparity maps for several window sizes and discuss which window
size works the best (or what are the tradeoffs between using different window sizes). How does the running
time depend on window size?
- Disparity range: what is the range of the scanline in the second image that should
be traversed in order to find a match for a given location in the first image? Examine the stereo
pair to determine what is the maximum disparity value that makes sense, where to start the search
on the scanline, and which direction to search in. Report which settings you ended up using.
- Matching function: try sum of squared differences (SSD), sum of absolute differences (SAD), and normalized correlation.
Discuss in your report whether there is any difference between using these functions, both
in terms of quality of the results and in terms of running time.
In addition to showing your results and discussing implementation parameters, discuss the shortcomings
of your algorithm. Where do the estimated disparity maps look good, and where do they look bad? What would be required
to produce better results? Also discuss the running time of your approach and what might be needed to make
stereo run faster.
Part 2 Extra Credit
-
Convert your disparity map to a depth map and attempt to visualize the depth map in 3D. Just pretend that
all projection rays are parallel, and try to "guess" the depth scaling constant. Experiment with
displaying a 3D point cloud, or computing a Delaunay triangulation of that point cloud.
-
Find additional rectified stereo pairs on the Web and show the results of your algorithm on these pairs.
-
Find non-rectified stereo pairs and rectification code on the Web and apply your algorithm to this data.
-
Implement multiple-baseline stereo as described in
this paper. Use
this data.
-
Try to incorporate non-local constraints (smoothness, uniqueness, ordering) into your algorithm.
You can come up with simple heuristic ways of incorporating these constraints, or try to
implement some of the more advanced methods discussed in the course (dynamic programming, graph cuts).
For this part, it is also fine to find code on the web.
Submission Instructions
You must upload the following files on Canvas:
- Your code in two separate files for part 1 and part 2. The filenames should be lastname_firstname_a5_p1.py and lastname_firstname_a5_p2.py. We prefer that you upload .py python files, but if you use a Python notebook, make sure you upload both the original .ipynb file and an exported PDF of the notebook.
- A report in a single PDF file with all your results and discussion for both parts following this template. The filename should be lastname_firstname_a5.pdf.
- All your output images and visualizations in a single zip file. The filename should be lastname_firstname_a5.zip. Note that this zip file is for backup documentation only, in case we cannot see the images in your PDF report clearly enough. You will not receive
credit for any output images that are part of the zip file but are not shown (in some form) in the report PDF.
Please refer to course policies on academic honesty, collaboration, late days, etc.